As a pre-med student, Francy Shu, MD, was close friends with an older couple from her church. Both in their 70s, “Aunt Julie” and “Uncle Jay” would invite her over for warm, home-cooked meals and good company. Though they were generally in good health, Uncle Jay had a tremor that caused pretty severe shaking in his hands.
One day, Aunt Julie tripped in the kitchen and broke her hip. She couldn’t get up, and Uncle Jay’s tremor prevented him from dialing a phone to get help. Helpless, he watched his wife bleed to death.
Since then, Dr. Shu, now a neurologist at UCLA Health, has been dedicated to preventing similar tragedies.
“Over the years, my study room at home has turned into a fall detection lab,” she said. Now, she’s developed an artificial intelligence-based fall detection system that can monitor an entire house at a fraction of the cost of existing systems.
Fall detection systems currently on the market can run in the thousands of dollars, making them inaccessible to many, especially seniors on a fixed income, Dr. Shu said.
In general, existing systems either incorporate wearable sensors or surveillance cameras. In the event of a fall, a computer sends a message to a predetermined contact, such as a family member, a neighbor or a medical emergency response team. The notified party can take action accordingly.
“We thought about wearable sensors,” Dr. Shu said, “but people forget to wear them, or forget to change the batteries.” Wearable systems can be uncomfortable and healthy people may not feel the need to wear them all the time.
Camera-based systems, on the other hand, have their own drawbacks. They require powerful computer processors to analyze 3-D image data and detect a fall – and can thus get expensive. “If the image is processed in color, every pixel has 16 million variables,” Dr. Shu said. Not only that, she said, they can’t reliably detect falls when multiple moving objects are in view or if the line of sight is blocked by furniture.
Dr. Shu partnered with her father, artificial intelligence expert Jeff Shu, to build a fall detection system that uses cameras but requires less computing power than existing image-based systems.
Dr. Shu published her design for the house-wide fall detection system in the journal Scientific Reports.
“Instead of analyzing the entire image, we extract the physical parameters to determine which ones are falls,” Dr. Shu said. “This method significantly reduces the amount of data. It allows us to monitor many cameras simultaneously at a very high frame rate.”
By analyzing the physics of falls, the researchers determined some of the crucial characteristics that can be used to distinguish true falls from daily activities. These include acceleration and movement patterns of the head and legs.
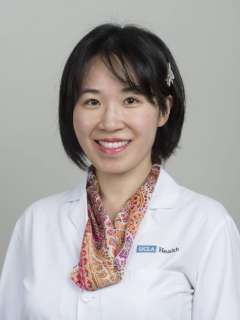
“Sometimes it was difficult to differentiate true falls from stunt performances, so we needed more information,” Dr. Shu said. In those cases, they increased the frame rate to identify more pertinent information, such as the abrupt loss of speed when hitting the ground, while also removing extraneous data.
Eventually, they trained the computer to characterize falls using very few pieces of data and without processing every pixel of the image. “You don’t need to know the trees are green; you don’t need to know a bird is flying by,” Dr. Shu explained.
To be useful, the system must distinguish between falls and similar movements, such as jumping, sitting on the floor or bending over to put on shoes.
At the same time, not all falls have the same physical profile. Tripping over an object on the floor causes a person to pitch forward, for instance, while fainting is more of a vertical collapse, and falling off a ladder requires the computer to understand the shift in height.
Dr. Shu trained the system on various patterns of activity, including six categories of falls and six common non-fall activities, at different angles, directions, distances and heights.
The timeline to get the system into production has been slowed by the pandemic, but Dr. Shu said that once production facilities are back in business, it won’t be long before the system is available.
Learn more about UCLA Health's Department of Neurology.
Caroline Seydel is the author of this article.