Integrative Biostatistics and Bioinformatics Core
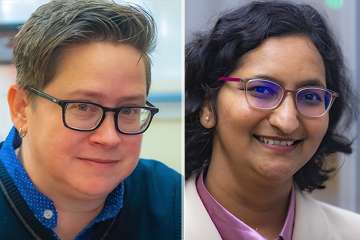
Combining state-of-the-art statistics with bioinformatic knowledge bases, we will provide services to support researchers and clinicians interested in integrating clinical and biological data (e.g. microbiota, metabolites, metagenomics, transcriptomics, epigenetics, multimodal neuroimaging data obtained from human and animal studies).
Integrative Biostatistics and Bioinformatics Core Director Jennifer S. Labus, PhD, and Co-Director Swapna Joshi, PhD
For more information, contact [email protected]
Services of the Integrative Biostatistics and Bioinformatics Core
- Assist with developing hypotheses, experimental design and data analytic plans using novel bioinformatics and biostatics approaches for ongoing studies, data mining and the development of new grant applications. Particular areas of expertise include:
- Advanced multimodal neuroimaging including regional brain morphometry (structural magnetic resonance imaging), functional (resting state and task-based fMRI) and anatomical connectivity (diffusion spectrum imaging), positron emission tomography (PET)
- Multi-omics microbiome assessment (e.g. 16S rRNA sequencing, shotgun metagenomics, metabolomics)
- Epigenetic and transcriptomics analyses
- Deliver state-of-the-art biostatistics and bioinformatics expertise for hypothesis testing of cross-sectional and longitudinal studies
- Linear and generalized linear models (e.g., logistic regression, random effects zero-inflated negative binomial models, log-linear models)
- Generalized estimation equations for correlated longitudinal data, longitudinal data (e.g., logistic regression, random effects zero-inflated negative binomial models, log-linear models), latent variable modeling, autoregressive cross-lagged models
- Perform systems biology and multi-omics integration analyses
- Apply data preparation/preprocessing for quality control including data cleaning, data profiling (i.e., normality, outlier detection, sparsity), data visualization, missing value analysis, transformation, normalization, integration of multiple datasets
- High-level pathway and network analysis to elucidate complex pathobiological patterns (e.g., graph theory-based association network analysis, co-inertia analysis, sparse generalized canonical correlation, graphical models, Bayesian networks)
- Supervised machine learning (e.g., sparse partial least squares, elastic net regression, boosting models)
- Unsupervised machine learning (e.g., ensemble clustering, artificial neural networks)
- Deep learning applications (e.g., deep neural networks, convolution neural networks, autoencoders)
- Assist with translational interpretation of analysis results of multi-omics data analysis obtained from human and animal studies
- Draft statistical sections for grant applications, study protocols and research
- Manuscripts