Recovering After Stroke: The Geometry of Motion
Dr. Ahmet Arac Makes Strides in Re-Gaining Movement Post-Stroke
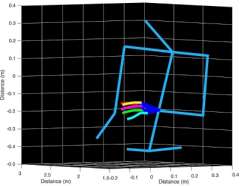
Fall 2018
How does the brain re-gain control of movements after stroke? How does the brain learn new skills in the first place? Are these two things related, and can learning be enhanced to improve recovery after stroke? These are the big questions asked by Dr. Ahmet Arac, a physician-scientist in the Neurorehabilitation Program in UCLA Neurology. Pursuing these questions requires scientific approaches across several disciplines, including brain imaging, rehabilitation care and artificial intelligence. Dr. Arac just completed his Neurology residency at UCLA, attaining a competitive research grant to dedicate time during the residency for research (an “R25” grant from the NIH) and now receiving another competitive NIH award, a “K08” award for new investigators to come onto faculty.
Motor skill learning is characterized by improvement in the speed and accuracy of new tasks. This “motor skill” does not always have to be a Grand Slam-winning serve in tennis or the marvelous finger movements of a piano virtuoso playing Rachmaninoff’s Piano Concerto No. 3. In fact, even simple motor behaviors such as reaching, when examined in detail, can be very difficult to define—how does one measure the elements in skilled reach to grasp and drink a cup?
Yet, to study how the body recovers after stroke, we have to be able to measure how we move our limbs. For example, during skilled reach, healthy people can sit back in a chair and use their shoulder limb muscles to brace the arm in support, and the arm is used for elevating, extending and grasping. After stroke, patients often lean in and use their shoulder limb muscles to help elevate and extend the arm, and do not recover their arm muscles for the elevating or extending. Clinically, this is important because simple and inexact tests of recovery after stroke, termed “motor impairment scores” (the Fugl-Meyer scale or the Action Research Arm Test) measure only the outcome of a complex movement (grasping the cup), but not the components. Kinematic analysis is the detailed description of movement patterns, literally the geometry of motion. Kinematic analysis reveals unforeseen information about the timing and typicality of movements. Kinematic analysis distinguishes true recovery—the stroke patient that is able to sit back and smoothly reach and grasp a cup—from compensation, when the arm and hand really cannot do this, so the patient leans forward and braces the body to force and suspend the arm. Both approaches lead to grasping a cup, but only the first is the efficient and reliable method that body used for all of its time prior to stroke. If our goal in the future of stroke rehabilitation is to develop treatments that stimulate true recovery, we have to be able to identify the difference between recovery and when the body compensates to accomplish the goal.
What quantitative methods can we use to perform kinematic analysis during motor skill learning and recovery after brain injury? Dr. Arac realized that he can use recent technological advances in computer science and machine learning fields to perform an analysis of joint and limb movement over time in a task to measure how patients are affected by stroke and subsequent recovery. This type of inter-disciplinary work brings together expertise in multiple fields, computer science and machine learning on one end, and neuroscience and clinical neurology on the other. This is innovative as for the first time, human movement can be defined in detail in its very natural form without external devices attached.
These techniques utilize advanced mathematical tools, including deep learning algorithms. Deep learning is a branch of the machine learning field and is the fundamental backbone of the current artificial intelligence revolution. It is the main mathematical algorithm behind self-driving cars, face and speech recognition systems, as well as natural language processing. By using these techniques, Dr. Arac can analyze the video recordings of patients performing certain tasks without the need for wearing any special equipment. He then determines the 3D positions of each joint based on these videos and establishes the 3D movement patterns in automated and unbiased ways. He can analyze the timing and typicality of the movements, define the richness and complexity of the naturally occurring motor behaviors, detect the changes that occur after neurological injuries, and track the recovery of these patients. This type of unbiased and automated analysis has the potential to identify structures in the data that are otherwise very hard to reveal with just direct visual observation. Artificial intelligence-guided kinematic analysis will revolutionize the way recovery is defined and clinically tracked, and help develop new interventions for stroke recovery.
In a similar manner, he developed similar tools to study the skilled motor behavior in laboratory mice. The advantage of conducting these studies in mice is that the availability of advanced brain recording and cellular and molecular imaging tools makes it possible to identify the underlying mechanisms (the brain cells themselves) engage in motor learning and recovery after stroke. We can measure the activity from hundreds of brain cells in different brain regions, and determine the mathematical relationship between the activity patterns of the brain cells and the fundamental kinematic elements of movement during motor skill learning and stroke recovery with rehabilitative training. We can then intervene with the molecular tools to identify the precise mechanisms.
Understanding the mechanisms of movement will ultimately lead to better therapies for stroke recovery. Dr. Arac expects that this approach with detailed mathematical analysis of behavior and the use of recording and manipulation techniques in the brain will generate fundamental knowledge about stroke recovery to inform new interventions that can reduce the burden of disability caused by stroke.
Next Story: A Neurophysicist; The Ultimate Border Crossing Incursion >