Bioinformatics & Perioperative Analytics
THE DIVISION OF BIOINFORMATICS AND PERIOPERATIVE ANALYTICS

At a fundamental level, high-quality data, and knowing how to interpret it, form the foundation of knowledge development. Our group in the UCLA DAPM Division of Bioinformatics & Perioperative Analytics strives to apply diverse Health Care data sources to drive innovation, deepen understanding of complex medical systems, and improve clinical outcomes. Our data sources range from the electronic health record to social determinants of health and are linked to databases with waveform and genomic repositories. Our tools range from traditional statistics and data discovery techniques to emerging analytics such as artificial intelligence and natural language processing. We collaborate regularly with other large institutions and are involved several national registries.
The ultimate goal of all of our efforts is applying technology to improve clinical care. With 42 million patients undergoing surgery, and nearly 6 million admitted to an intensive care unit annually, the perioperative and critical care periods are critical risk periods for patients. Our goal is to develop high-quality data sources, pioneer usage of advanced analytic techniques, and implement real-time risk tools that improve patient care.
UCLA CENTER OF EXCELLENCE FOR PERIOPERATIVE ANESTHESIA
We are designated by the health center as a Center of Excellence for Perioperative Anesthesia. As such, we are dedicated to advancing the field of perioperative and critical care. As a leading institution in perioperative medicine, our center focuses on providing comprehensive care, utilizing advanced techniques, conducting research and innovation, offering education and training programs, fostering collaboration and networking, and prioritizing patient-centered care. With a commitment to excellence, our center strives to optimize patient outcomes and enhance the quality of perioperative anesthesia care.
- MISSION: The Division of Bioinformatics and Analytics is committed to leveraging technology to improve delivery of patient care throughout the acute care period, conducting and supporting innovative research, and cultivating a community that is skilled in interpreting and utilizing healthcare data.
“Improving patient care bit by bit.”
- VISION: We aspire to significantly increase the value, innovation, and partnerships of our Bioinformatics division. Our goal is to deepen involvement from trainees to faculty, utilizing technology to revolutionize the practice of medicine, and achieving greater prominence both nationally and within the health system.
- VALUES: Our foundation is built on teamwork, communication, trust, and innovation. We promote career development, value the quality of our work, and strive for continuous improvement in healthcare informatics.
Our scientific approach is based on the following expertise:
Over the years, we've led the way in developing and clinically validating a wide range of healthcare data sources. This includes diverse data types like electronic health records (EHRs), as well as external databases such as echocardiogram and blood bank systems. We've also incorporated geographically-oriented data into our research, providing valuable insights into social determinants of health and their impact on perioperative outcomes. Through our commitment to innovation and collaboration, we're expanding the scope and depth of healthcare data available for research and clinical use, driving advancements in perioperative medicine and patient care.
We have been innovators in application of artificial intelligence algorithms and advanced analytics. Our innovative approach encompasses harnessing the capabilities of AI to analyze vast and complex datasets, such as electronic health records and medical imaging, to derive actionable insights for patient care. Through the development and implementation of AI-driven predictive models, we aim to enhance risk assessment, optimize treatment strategies, and improve patient outcomes across the perioperative continuum.
Our scope includes integration of advanced clinical decision support systems and digital quality improvement methodologies. Several instances of our work has demonstrated significant clinical benefit of innovative clinical decision support systems.
And finally, we collaborate with other departments within UCLA to provide analytic support.
We believe collaboration among clinicians, computer scientists, and individuals from diverse backgrounds is essential for leveraging diverse perspectives, domain knowledge, and technical skills to develop and implement effective informatics solutions that directly impact patient care, outcomes, and healthcare delivery.
CLINICAL INNOVATIONS
Ten years ago, the UCLA Perioperative Data Warehouse was established with the aim to advance perioperative research and clinical practice within UCLA Department of ANestheisology and Perioperative Care. At its core, a data warehouse serves as a centralized repository, harmonizing diverse and sometimes unstructured data into a cohesive and accessible format. This initiative was driven by the recognition of the immense potential inherent in combining disparate perioperative variables, enabling researchers and clinicians alike to glean insights and make informed decisions with unprecedented efficiency. By streamlining data accessibility and organization, the UCLA Perioperative Data Warehouse empowers end users to extract meaningful information, leading to advancements in perioperative care, research, and patient outcomes. Our research ranges from investigating the impact of preoperative risk factors on postoperative outcomes to developing predictive models for perioperative complications. We pull data from diverse sources, including the electronic health record, external databases like echocardiogram and blood bank systems, and geographically-oriented datasets, allowing for an innovative and comprehensive exploration of perioperative factors and outcomes.
SIGNIFICANT PUBLICATIONS
- Hofer IS, Gabel E, Pfeffer M, Mahbouba M, Mahajan A. A Systematic Approach to Creation of a Perioperative Data Warehouse. Anesth Analg. 2016 Jun;122(6):1880-4.
- M Willingham, S Al Ayoubi, M Doan, T Wingert, J Scovotti, K Howard-Quijano, JP Neelankavil. Preoperative Diastolic Dysfunction and Postoperative Outcomes after Noncardiac Surgery. J Cardiothorac Vasc Anesth. 2020 Mar;34(3):679-686. PMID: 31759861
- J Kim, A Wu, T Wingert, J Scovotti, T Grogan, W Kratzert, JP Neelankavil. Frequency and outcomes of elevated perioperative lactate levels in adult congenital heart disease patients undergoing cardiac surgery. J Cardiothorac Vasc Anesth 2020 Oct;34(10):2641-2647. PMID: 32139342
- T Wingert, T Grogan, M Cannesson, A Sapru, W Ren, I Hofer. Acute Kidney Injury and Outcomes in Children undergoing Noncardiac Surgery: a Propensity-matched Analysis. Anesth Analg 2021 Feb 1;132(2):332-340. PMID: 32739953.
- M Hernandez-Morgan, JP Neelankavil, T Grogan, B Hong, T Wingert, E Methangkool. Preoperative Anemia as a Risk Factor for Postoperative Outcomes in Patients Undergoing Lung Transplantation. J Cardiothorac Vasc Anesth 2021 Aug;35(8):2311-2318. PMID: 33293217.
Our team has been at the forefront of innovative research in machine learning and its applications in perioperative medicine. We've pioneered the development of predictive models for various perioperative outcomes, including mortality, postoperative ventilator requirements, risk stratification, and leveraging digital phenotyping for novel preoperative risk stratification. We have been at the forefront of applying advanced analytics such as machine learning (ML) and artificial intelligence (AI) in perioperative medicine. Our research has not only improved risk assessment and patient stratification but has also enhanced decision-making processes in perioperative care. By harnessing the power of ML and AI, we strive to reshape the landscape of perioperative medicine, paving the way for more personalized and effective patient management strategies.
SIGNIFICANT PUBLICATIONS
- Gabel E, Hofer IS, Satou N, Grogan T, Shemin R, Mahajan A, Cannesson M. Creation and Validation of an Automated Algorithm to Determine Postoperative Ventilator Requirements After Cardiac Surgery. Anesth Analg. 2017 May;124(5):1423-1430.
- Lee CK, Hofer I, Gabel E, Baldi P, Cannesson M. Development and Validation of a Deep Neural Network Model for Prediction of Postoperative In-hospital Mortality. Anesthesiology. 2018 Apr 17.
- Hofer IS, Cheng D, Grogan T, Fujimoto Y, Yamada T, Beck L, Cannesson M, Mahajan A. Automated Assessment of Existing Patient's Revised Cardiac Risk Index Using Algorithmic Software. Anesth Analg. 2018 May 25.
- E Rahmani, R Schweiger, L Shenhav, T Wingert, I Hofer, E Gabel, E Eskin, E Halperin. Bayes CCE: a Bayesian framework for estimating cell-type composition from DNA methylation without the need for methylation reference. Genome Biol. 2018 Sep 21;19(1):141. PMID: 30241486
- Hofer IS, Lee C, Gabel E, Baldi P, Cannesson M. Development and validation of a deep neural network model to predict postoperative mortality, acute kidney injury, and reintubation using a single feature set. NPJ Digit Med. 2020 Apr 20;3:58. doi: 10.1038/s41746-020-0248-0. PMID: 32352036; PMCID: PMC7170922.
- Mišić VV, Gabel E, Hofer I, Rajaram K, Mahajan A. Machine Learning Prediction of Postoperative Emergency Department Hospital Readmission. Anesthesiology. 2020 May;132(5):968-980.
- T Wingert, C Lee, M Cannesson. Machine learning, deep learning, and closed loop devices – anesthesia delivery. Anesthesiol Clin 2021 Sep;39(3):565-581. PMID: 34392886.
- P Laferrière-Langlois, F Imrie, MA Geraldo, T Wingert, N Lahrichi, M Schaar, M Cannesson. Novel Preoperative Risk Stratification Using Digital Phenotyping Applying a Scalable Machine-Learning Approach. Anesth Analg. 2023 Dec 5. PMID: 38051671.
- Stocking JC, Taylor SL, Fan S, Wingert T, Drake C, Aldrich JM, Ong MK, Amin AN, Marmor RA, Godat L, Cannesson M, Gropper MA, Utter GH, Sandrock CE, Bime C, Mosier J, Subbian V, Adams JY, Kenyon NJ, Albertson TE, Garcia JGN, Abraham I. A LASSO-derived predictive model for postoperative respiratory failure in a heterogeneous adult elective surgery patient population. CHEST Critical Care (2023).
- Zarrin D, Suri A, McCarthy K, Gaonkar B, Wilson B, Colby G, Freundlich R, Macyszyn L, Gabel E. Machine Learning Predicts Cerebral Vasospasm in Subarachnoid Hemorrhage Patients. Res Sq [Preprint]. 2024 Feb 5:rs.3.rs-3617246.
The UCLA Bioinformatics Division enhances healthcare through Clinical Decision Support (CDS) daily. So much of the pathways and workflows that our providers have become accustomed to are executed via CDS alerts through the EHR and email. This works because of our well-established collaboration with the Epic build team the has made the integration of our PDW system and the EHR provider experience seamless. We've implemented CDS in PONV management, hypotension prevention strategies, and developed a machine learning mortality prediction, showcasing our innovative approach to patient care. Our review articles further demonstrate our expertise and commitment to advancing CDS. Lastly, our division is running a clinical trial that is fully automated, facilitating patient enrollment without human coordinators, underscoring our pioneering use of technology in research.
Expanding upon the administrative applications of data within the UCLA Bioinformatics Division, we've modernized hospital and departmental management. This encompasses generating precise financial reports via excel and tableau dashboard to drive efficiency, optimizing OR utilization alongside strategic staffing to enhance operational capacity, and advancing quality improvement initiatives through robust QI/QA reporting systems. We're also report on staffing ratios for balanced workload distribution, crafting tailored departmental process measures for performance benchmarking, and innovating with an automated system for triaging preoperative patients, thereby streamlining preop clinic processes. These data-driven strategies significantly contribute to a elevated departmental data standards.
STAFFING OF THE DIVISION

Eilon Gabel, MD
As the Director of Operations, Dr. Gabel’s leadership and vision steer the division towards innovative research and application of bioinformatics in improving patient care. Dr. Gabel’s recent contributions include research on the development and validation of deep neural network models for predicting postoperative outcomes using electronic health record data. This work showcases the potential of machine learning in enhancing patient safety and care quality.

Theodora E. Wingert, MD
Dr. Wingert leads the division’s research efforts, focusing on leveraging data analytics and machine learning to predict and improve perioperative outcomes. Her work emphasizes the importance of bioinformatics in advancing personalized medicine and enhancing clinical decision-making processes.
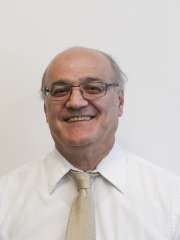
Valiollah Salari, PhD
Dr. Salari oversees our perioperative data warehouse environment, fostering high-level collaborations with the health system to ensure the effective operation of our data systems. Additionally, he leads our division's efforts in implementing machine learning solutions to enhance our analytical capabilities.
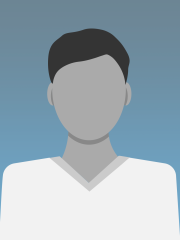
Stephen Murray
Stephen leads our departmental quality informatics initiatives, focusing on integrating various systems and platforms to streamline data collection and analysis processes. He also manages collaborations with external vendors, such as Qualtrics surveys, to improve our data gathering methodologies.
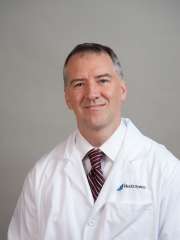
James M. Moore, MD
Dr. Moore plays a vital role in advancing our electronic medical records system, leveraging his expertise as a physician informaticist to optimize clinical workflows and enhance patient care delivery.
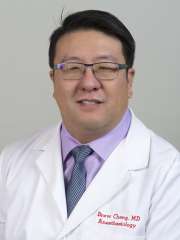
Drew S. Cheng, MD
Dr. Cheng specializes in extracting high-quality research data to support our clinical investigations within UCLA. Additionally, he oversees the management of the multi-center perioperative operating group (MPOG) registry, facilitating collaborative research efforts across our institution.

Jennah Varela
Jennah leads our project management efforts, ensuring the successful execution of initiatives within our division. As a skilled Tableau analyst, she also contributes to data visualization and analysis, providing valuable insights for decision-making processes.
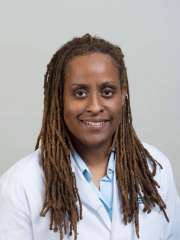
Tiffany M. Williams, MD, PhD
Dr. Williams, MD, PhD, is a distinguished member of our team, serving as the Lead Bioinformatics Scientist. Her dual qualification in medicine and bioinformatics enables her to spearhead innovative approaches in personalized medicine and genomics. Dr. Williams’s research focuses on integrating bioinformatics tools with clinical insights to dissect complex biological datasets, aiming to unveil novel diagnostic markers and therapeutic strategies.
Connect with us
For questions or more information on the Division of Bioinformatics & Perioperative Analytics, please email Jennah Varela at [email protected]. For inquires regarding the Fellowship in Perioperative Bioninformatics, we invite you to explore the webpage found here.
Follow us on social media: Instagram: @ucla_anesthesiology & Twitter/X: @UCLAAnes