Dr. Wayne G. Brisbane Lab
Wayne G. Brisbane, MD
Assistant Professor
UCLA Department of Urology
Dr. Brisbane’s research focuses on novel image-guided diagnosis and treatment of prostate cancer. His lab utilizes Micro-Ultrasound, MRI, and PSMA PET in combination with machine learning to improve the diagnostic accuracy for identifying prostate cancer. His work in Micro-ultrasound imaging is funded by the Prostate Cancer Foundation and the Phase One Foundation.
After skin cancer, prostate cancer is the most widely diagnosed cancer in men, with nearly 1 in 8 males diagnosed during their lifetime (Siegel et al., 2023). Tissue acquisition remains the standard for a prostate cancer diagnosis; over 1 million prostate biopsies are performed annually in the United States (Loeb et al., 2011).
The introduction of MRI enables improved cancer visualization compared to a systematic biopsy performed in a blind fashion (Elkhoury et al., 2019). However, many tumors (35-45%) remain invisible to MRI imaging (Ahmed et al., 2017; Johnson et al., 2019). Therefore, guideline organizations recommend MRI-targeted and systematic biopsies to avoid missing MRI-invisible tumors (Bjurlin et al., 2020; Mottet et al., 2021). Our group has demonstrated that this strategy results in oversampling benign prostate tissue. Only 12% of biopsy cores return with ≥ Grade Group 2 prostate cancer, and 90% are within 1 cm of the MRI target (Brisbane et al., 2022). Additionally, work out of the NCI identified that MRI-missed cancer is likely the result of fusion and interpretation errors (Williams et al., 2022).
Our lab has three goals:
- Refine imaging to remove the need for systematic biopsy and eventually biopsy altogether.
- Understand the biology of imaging invisible prostate cancers.
- Utilize imaging to define tumor boundaries for treatment.
Goal 1: Refine imaging to remove the need for systematic biopsy and eventually biopsy altogether.
Our lab has demonstrated that the majority of prostate cancer can be identified within 1 cm of MRI lesions (Figure 1). Only 12% of biopsy cores return with ≥ Grade Group 2 prostate cancer, also known as clinically significant prostate cancer.
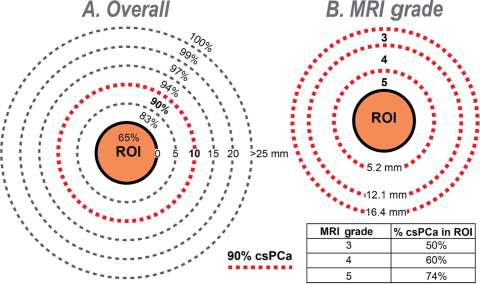
Figure 1: Diagrams showing (A) overall cumulative distribution of biopsy cores containing csPCa (% of total) in and around MRI lesion (region of interest, ROI) and (B) relationship of MRI grade on width of band (penumbra) containing 90% of csPCa. Note that (A) 90% of csPCa cores are found within a 10-mm radius from ROI surface (dashed red line), but only 65% are within ROI; and (B) width of the 90% penumbra (mm) is inversely related to MRI grade. Inset table shows the relationship of MRI grade with % csPCa in ROI (N = 3552 cores containing csPCa from 927 men). (A) Percent csPCa (95% CI) within boundary—within ROI: 65% (63–67%); 5 mm: 83% (81–84%); 10 mm: 90% (89–91%); 15 mm: 94% (93–95%); 20 mm: 97% (96–98%); and 25 mm: 99% (98–99%). (B) Percent csPCa (95% CI)—grade 3: 50% (46–54%); grade 4: 60% (57–64%); and grade 5: 74% (71–76%). CI = confidence interval; csPCa = clinically significant prostate cancer; MRI = magnetic resonance imaging; ROI = region of interest.
A possible solution to the current paradigm of oversampling to compensate for registration and MRI imaging error is the introduction of Micro-Ultrasound. Micro-Ultrasound images the prostate at 29 mHz compared to 9 mHz of conventional ultrasound (Figure 2). Micro-Ultrasound produces near histologic level imaging, with resolution down to 70 microns (Klotz, 2020). Initial work from our group demonstrated better tumor volume predictions with Micro-Ultrasound than MRI (Brisbane et al., 2021); prior comparisons of Micro-Ultrasound vs. MRI accuracy showed equivalent cancer detection (Klotz et al., 2021; Sountoulides et al., 2021). Analogous to the PI-RADS score for MRI, Micro-Ultrasound users score lesions based on acoustic features and assign a PRI-MUS score (Prostate Risk Identification Using Micro-Ultrasound) (Ghai et al., 2016). We are currently utilizing MRI + Micro-Ultrasound for biopsy to understand better how imaging synergy can prevent the need for systematic biopsy without compromising accuracy.
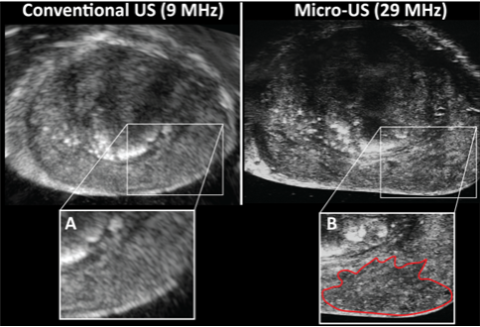
Figure 2: Axial imaging of an identical prostate location using conventional ultrasound with invisible tumor (A), and Micro-Ultrasound with a mottled hypoechoic area (PRI-MUS 4) representing 4+3 prostate cancer (B).
Goal 2: Understand the biology of imaging invisible prostate cancers
MRI and Micro-Ultrasound utilize different physical mechanisms for the visualization of prostate cancer (Dinh et al., 2016; Ghai et al., 2016). Work at UCLA in MRI has demonstrated that visible lesions are associated with aggressive genomic and proteomic expression (Bauman et al., 2020; Chua et al., 2017; Houlahan et al., 2019). However, work by Dr. Brisbane’s group has demonstrated that Micro-Ultrasound visibility modifies the cancer detection rate of MRI lesions (Table 1)(Ashouri et al., 2023). It is likely that a the MRI genomic and proteomic signature is more complex than visible vs. invisible. We hypothesize that Micro-Ultrasound visibility can stratify the MRI visible genomic signature. Additionally, we hypothesize that there are distinct proteomic and genomic expressions for MRI invisible but Micro-Ultrasound visible tumors. We are working with Paul Butros, PhD to delineate the genomic signatures for tumor visible and invisible cancers.
Cancer Detection Rate Stratified by PI-RADS and PRI-MUS (N=101)
|
PI-RADS 5 |
PI-RADS 4 |
PI-RADS 3 |
PI-RADS 1-2 |
PRI-MUS 5 |
94.4% |
100% |
- |
- |
PRI-MUS 4 |
73.9% |
45.4% |
22.2% |
- |
PRI-MUS 3 |
50.0% |
50.0% |
100% |
- |
PRI-MUS 1-2 |
25.0% |
27.3% |
13.3% |
0.0% |
Table 1: CDR of PI-RADS lesions based on the concomitant PRI-MUS score. Even among PI-RAD 5 lesions, the PRI-MUS score greatly modified cancer risk. ‘-‘ indicates that no patients met the PI-RADS and PRI-MUS criteria.
Goal 3: Utilize imaging to define tumor boundaries for treatment.
Understanding the location of prostate cancer within the gland is critical for treatment, including surgery, radiation, and emerging treatments such as focal therapy. We employ multiple imaging modalities compared to final pathology and AI algorithms to predict tumor extent.